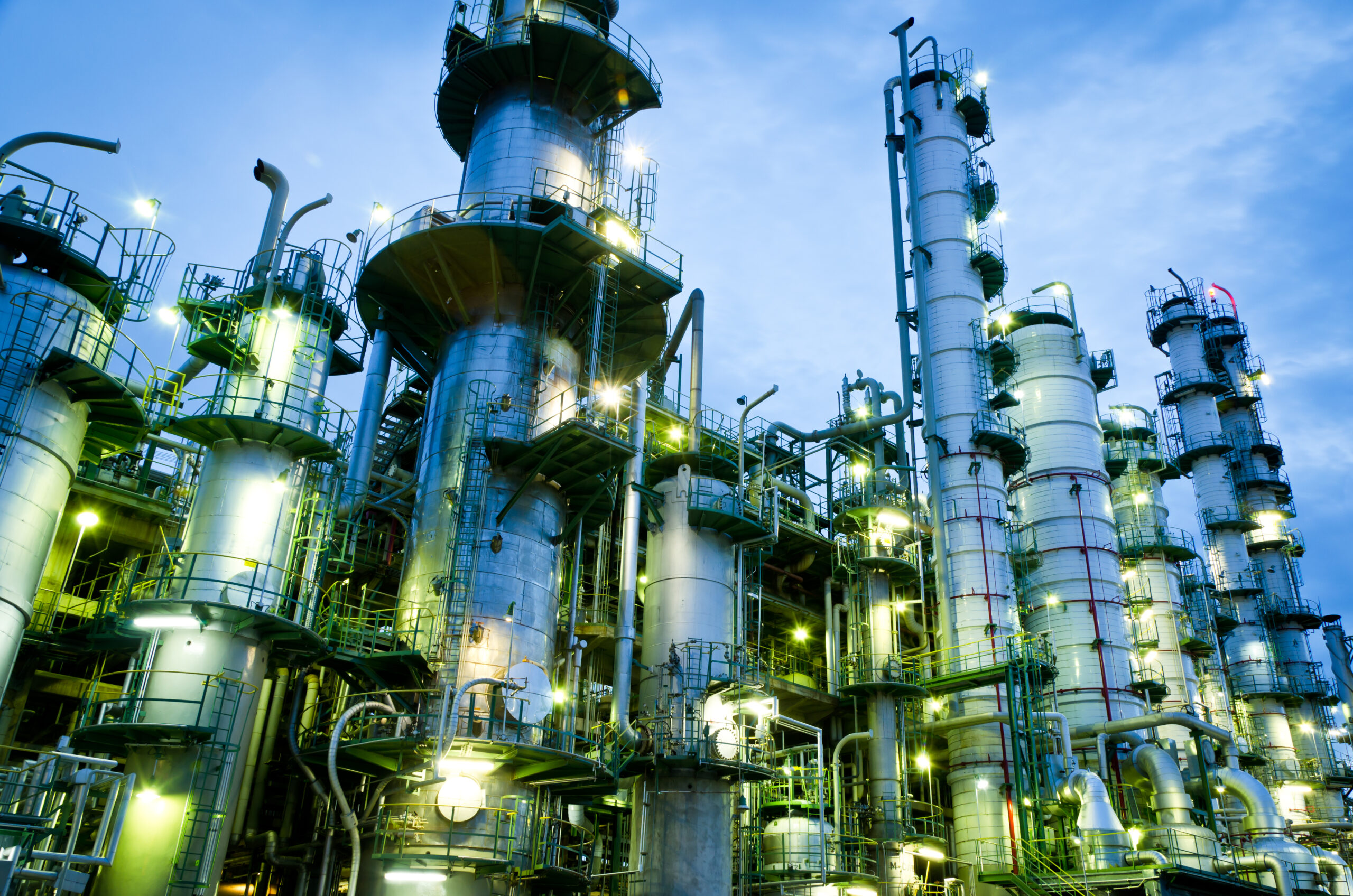
Predicting Product Properties Using Reactor Data
Challenge
In the chemicals industry, it is critical to control the finished product properties (such as product density, viscosity and reagent content) to maximize product quality, and as a result, profitability. Chemicals manufacturers test their finished products, placing them into different quality ranges based on how they perform in a series of laboratory tests. The highest quality product sells for the highest price, so maximizing the amount of the highest quality product will maximize the revenue of a particular process unit.
Lab testing can be labor and time-intensive, with the sampling rate typically in the range of hours or days. When a batch receives a failing quality grade, all of the material produced since the last passing result is typically downgraded to avoid sending low-quality material to customers as part of a high-quality material order.
When a bad result is received in a finished product sample during steady-state operation, the material currently in processing can be expected to yield a failing test result also. There have not historically been any software tools that are user-friendly enough to give process engineers and operators the ability to do the advanced data cleansing and multivariate modeling required to produce an accurate prediction of what a final product disposition will be based on what is going on in an upstream portion of the process.
A large-scale specialty chemicals company was looking for a way to minimize the profit loss associated with product downgrades. Developing a predictive model to reliably and accurately forecast product properties based on conditions in an upstream portion of the process would enable companies to make immediate process adjustments, minimizing the significant margin loss of product downgrades.
Solution
A large-scale polymer producer used these techniques to create a predictive model of a polymer product viscosity based on data from some upstream sensors in the reactors. Using Seeq advanced analytics software, the chemical company’s subject matter experts (SMEs) can now access advanced predictive modeling analysis. With the ability to easily shift signals forward and backward in time, users can adjust for process lag, or residence time between steps in a processing unit. This allows users to understand what types of upstream operating conditions yield the best production outcomes. Seeq’s point-and-click Prediction tool enables modeling of critical product quality parameters based on related variables upstream in the process. This model provides the consumers of the data with an estimate of the future quality disposition of the material currently in process in an upstream piece of equipment.
This analysis uses reactor temperature, monomer conversion and modifier feed rate to create a predictive model of product viscosity in a polymer process. Cleansed, delayed upstream signals are used as inputs into Seeq’s Prediction tool. The SMEs use Seeq’s Boundaries and Deviation Search tools to add quality specification limits to the viscosity parameter and identify time periods when the actual signal fell outside those bounds. Seeq formula was used to identify periods of time when the model accurately predicted the direction in which the actual viscosity deviated from target. These calculations were rolled up into a Scorecard to quantify the potential credits to be captured by employing a process control strategy based on predictive modeling.
Results
The model can predict historical product-quality excursions with significant reliability (r2 > 0.9). Using the model for near-real time quality control rather than traditional feedback methods has the opportunity to reduce product margin losses by more than $1,000,000 annually on a unit with an average production rate around 40,000 pounds per hour and a variable margin difference of $100/mT between on-specification and off-specification product.
Having this early insight into the quality outcome of upstream production allows process engineers to make proactive process adjustments, keeping the product quality parameter within the allowable range.
They compared the modeled viscosity signal with the actual lab results over the past couple years and found that during 82% of historical quality excursions, the model had accurately predicted an upset before it occurred. By shifting their product quality control strategy to making proactive reactor parameter adjustments based on the viscosity prediction, the company was able to improve the amount of low-quality product by greater than 50%, resulting in an increase in unit profitability of more than $600,000 per year.
Data Sources
- Sensor data from a Process Data Historian (OSIsoft PI, Honeywell PHD, Aspentech IP21, etc.).
- Analytical data from a Laboratory Information Management System (LIMS).
Data Cleansing
- Agile Filter applied to remove sensor noise from all model input signals.
- Time delays were applied to shift signals forward in time to account for process lag.
Calculations and Conditions
- Value Search, Periodic Condition, Composite Condition, Prediction, Signal from Condition, Scorecard Metric, Boundaries, Deviation Search, Histogram, Seeq Formula.
Reporting and Collaboration
An Organizer Topic summarizing the challenge and approach was created for sharing results of the analysis with the team. The Organizer Topic includes:
- A table of monthly financial opportunities is listed first to highlight the large amount of annual credits that could be captured by deploying this type of model.
- Scatterplots to visualize overall model fit and a histogram to see fit by grade.
- Chain View is used to highlight model behavior during actual product viscosity deviations.
- A table summarizing the last three quality excursion events.