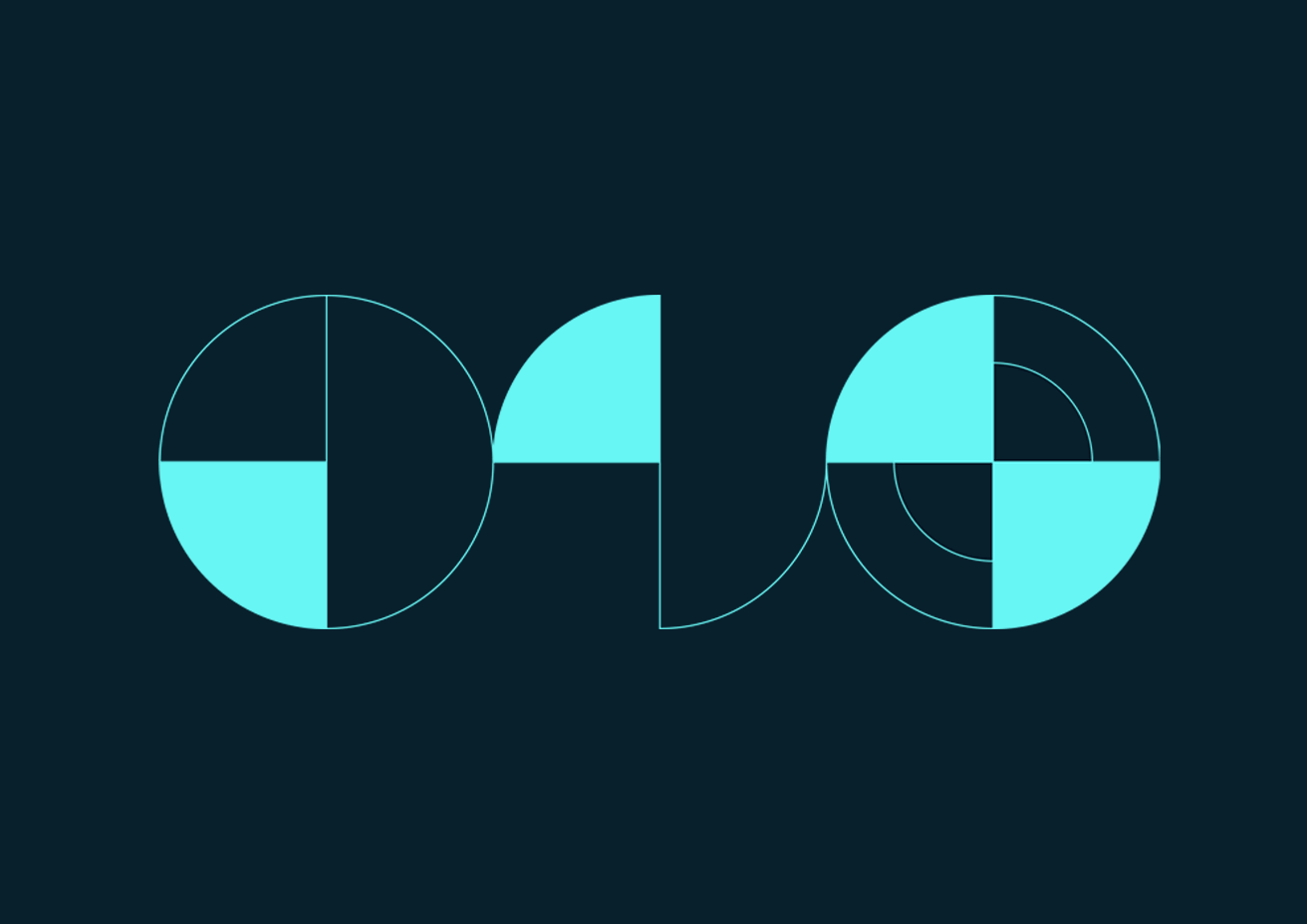
Unlocking Sustainability: Analytics in Semiconductor Facilities
Semiconductor fabs have long been on the leading edge of smart manufacturing with impressive, fully automated systems, approaching lights–out manufacturing. While manufacturing technology has taken huge leaps forward, the adoption of modern data analytics tools in these facilities has lagged. With the increasing focus on manufacturing sustainability and a constant increase in chip demand, companies are facing challenges to make more faster, while also reducing their impact.
A McKinsey report found that the majority of semiconductor manufacturers aim to be Net Zero by or before 2050, with Scope 1 and 2 emissions (emissions from the direct manufacture as well as energy purchased and steam/cooling water demands) accounting for 80% of all emissions associated with chip manufacture (McKinsey study). To accomplish net-zero emissions in chip manufacturing, companies need to enable their most important asset – their people – by providing purpose-built time series analytics tools to empower every subject matter expert (SME) to optimize processes, reduce resource use, and bring down costs.
Where are we now?
The current status quo for data analysis in the semiconductor space is a tabular environment like Excel. In most cases, an SME must first download thousands of rows of data from their process historian or data archive to analyze it in Excel, arduously matching time stamps and cleansing data before a simple analysis can be done. But once it’s complete, the analysis is already out of date. Homegrown analytics systems take on that load at some manufacturing sites, but these are often slow and cumbersome, and don’t keep pace with the rate of innovation. This leaves a huge amount of untapped potential, resulting in millions of dollars in lost optimization left on the table every day.
With Seeq, users can access their data seamlessly with pre-built connectors to most data sources and use purpose-built time series data analytics tools and the Seeq AI Assistant in a low-code, no-code environment. They can cleanse, contextualize, model, and iterate quickly – testing hypotheses on the fly. Once they have created an analysis, it continues to update with near real-time data and can even be scaled to similar equipment with the click of a button.
A path forward for facilities teams
So, where to start? How about the processing area in a semiconductor space that has long been overlooked – the facilities or fab support groups. These groups and the equipment they manage play a vital role in sustainable manufacturing and reducing manufacturing costs, but are often bypassed for new technologies. In fact, the operations associated with the facilities space account for up to 30% of fab operating expenses, and could be cut by 20-30% through changes in plant operation methods alone (McKinsey Study) This makes them uniquely poised to help drive down costs and make chip manufacturing more sustainable.
Much of the equipment used in the facilities space is “older technology:” unit operations like heat exchangers, pumps, filters, and even ion exchange beds that have been around for decades. These systems are considered well-understood and have not been the target for newer analytical techniques, from simple regression-based modeling to more advanced machine learning (ML). Data analysis for this critical equipment is often done offline in Excel or in a generic asset performance management software suite prone to over-alerting, where it quickly gets ignored. But sub-optimal performance of this critical equipment should not be overlooked, it can mean higher energy costs, equipment degradation, and unplanned downtime.
Let’s zoom in on one system within the facilities group: the HVAC system. HVAC systems employed by semiconductor and high-tech manufacturers are monolithic; they use huge amounts of energy and are sized on a scale almost unheard of in any other industry. The HVAC system is vital to the fab’s functioning and wafer yield, but are often monitored offline in Excel. Using Seeq, SMEs can get rid of their clunky spreadsheets and deploy predictive energy models using point-and-click tools in Seeq. These models can be developed and applied to the entire HVAC system in hours. With these analytics, SMEs can understand the current performance of the equipment and create email alerts at the first sign of degradation. This helps schedule optimal maintenance windows, reduce excessive energy use, and prevent fab impacts.
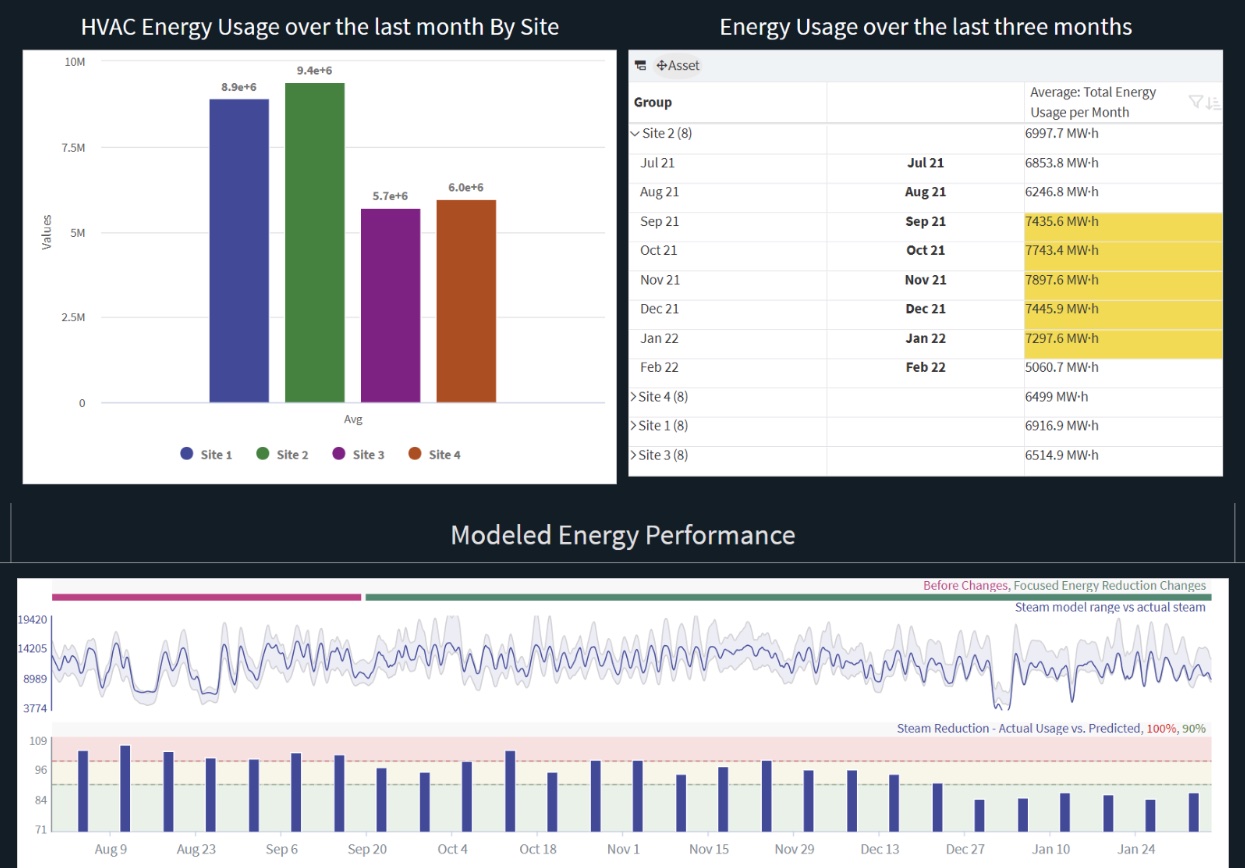
On top of enhancing workflows for fab personnel beyond tabular analytics, Seeq continues to innovate and is working to bring AI and ML into the hands of end users who are best poised to take advantage of these newer technologies for the greatest improvement. Add-on calculations are enabling data science groups to extend Seeq’s base capabilities for specific needs using Python, whether for process control or more advanced ML. In both cases, the SME and the data scientist can work together to build the best outcome, and then, using Seeq, scale that outcome from one asset to all applicable assets.
At a global wafer manufacturer, a group of process cooling water engineers leveraged the Seeq AI Assistant to perform data cleansing and contextualization in Seeq. The AI Assistant lowered the barrier to entry to advanced analytics, allowing the SMEs to converse in natural language to learn how to complete their analysis and even leverage the assistant to apply analytics for them. With the data for a fleet of cooling water pumps cleansed and contextualized, they shared this information with their data science team, seamlessly passing the data through Seeq for ML model building. The data science team developed ML algorithms on the data and deployed them directly back into Seeq. The cooling water team then scaled out the ML models to all pumps in the system for advanced anomaly detection, greatly reducing the risk of a failure and a fab–down scenario.
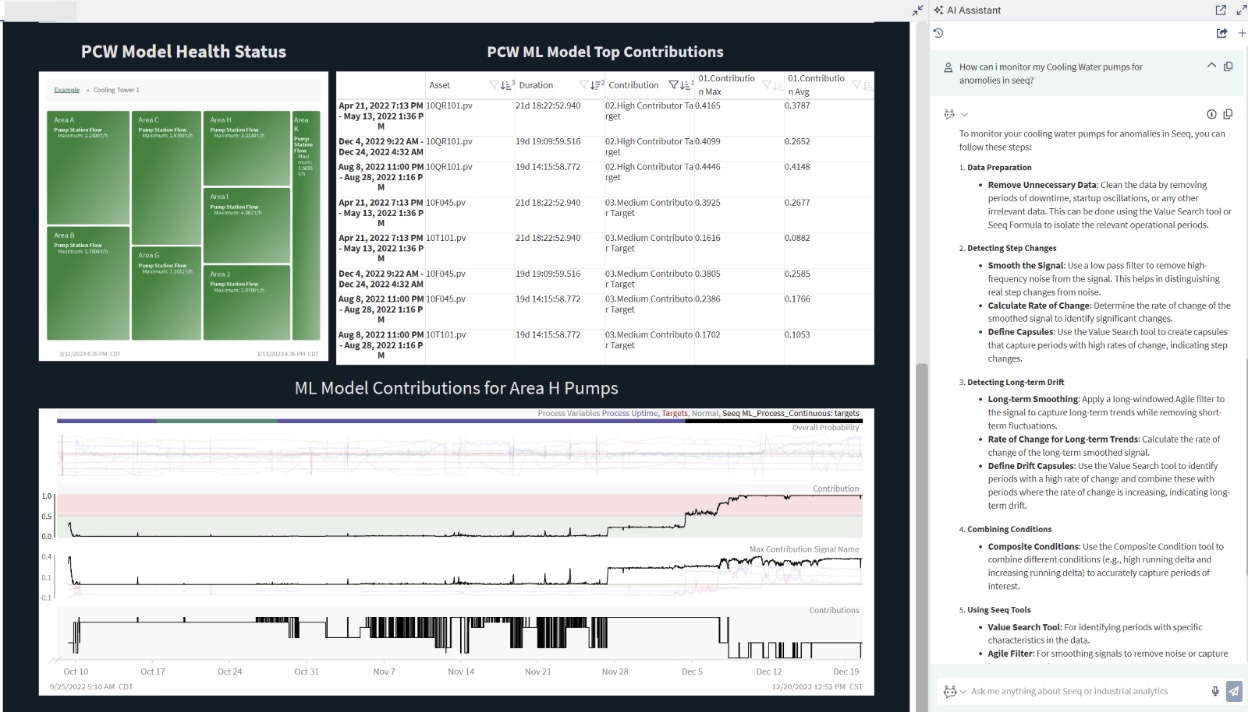
Improve Your Outcomes
Using a purpose-built advanced analytics platform like Seeq, SMEs can quickly identify and catch events before they escalate into an excursion and drive continuous improvements more effectively. With SMEs leading the way to reduced energy and utility consumption, semiconductor manufacturers can make large strides toward reaching their net-zero goals.
If you are ready to discuss how Seeq can help your organization reach its net-zero goals, please contact us to speak with one of our industry experts and schedule a demo today.