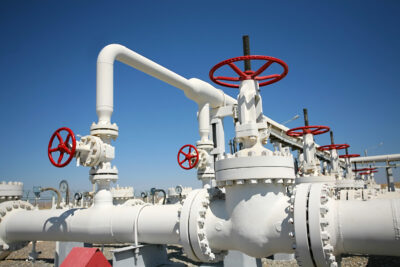
Detect Valve Erosion and Predict Failure
Challenge
In the process manufacturing industries, it’s difficult to characterize erosion by just monitoring the univariate process variables around valves. This lack of visibility leads to failure incidents, which are a risk to worker health, plant safety, and the surrounding environment. These incidents can also quickly lead to additional costs due to unplanned maintenance and production downtime.
Solution
Using Seeq, manufacturers can implement a condition-based monitoring analysis to monitor valve health across their fleet and accurately estimate time-to-failure. Subject matter experts can utilize historical failure data to create a predictive maintenance forecast to preemptively detect valve performance issues before failures occur. Valve health is determined by monitoring its performance with first principles analytics (valve Cv), which leads to identifying bad actors.
Results
The likelihood of unexpected valve failure is significantly reduced, saving costs due to lost production and unplanned maintenance. Implementing this analysis will also decrease health, safety, and environmental risks due to loss of containment if any valve fails.
Data Sources
- Process Data Historian: OSIsoft PI
- Maintenance Records: SAP
Data Cleansing
- Use Agile Filter to smooth noisy data
- Remove production downtime periods from analysis data set
Calculations and Conditions
- Formula to calculate first principles parameters: Operating Cv (actual) versus Theoretical Cv (expected) based on Flow Rate, Valve Position, and Pressure Drop across valve; also calculate Delta Cv
- Boundaries and Value Search to find areas where Operating Cv is > +/- 10% if Theoretical Cv
- Prediction to create a regression model to estimate Delta Cv behavior into future based on past historical failures (training window)
Reporting and Collaboration
- Prediction monitoring Trend in Organizer Topic
- Tables showing Estimated Failure Date / Recommended Maintenance Date / Time-to-Failure