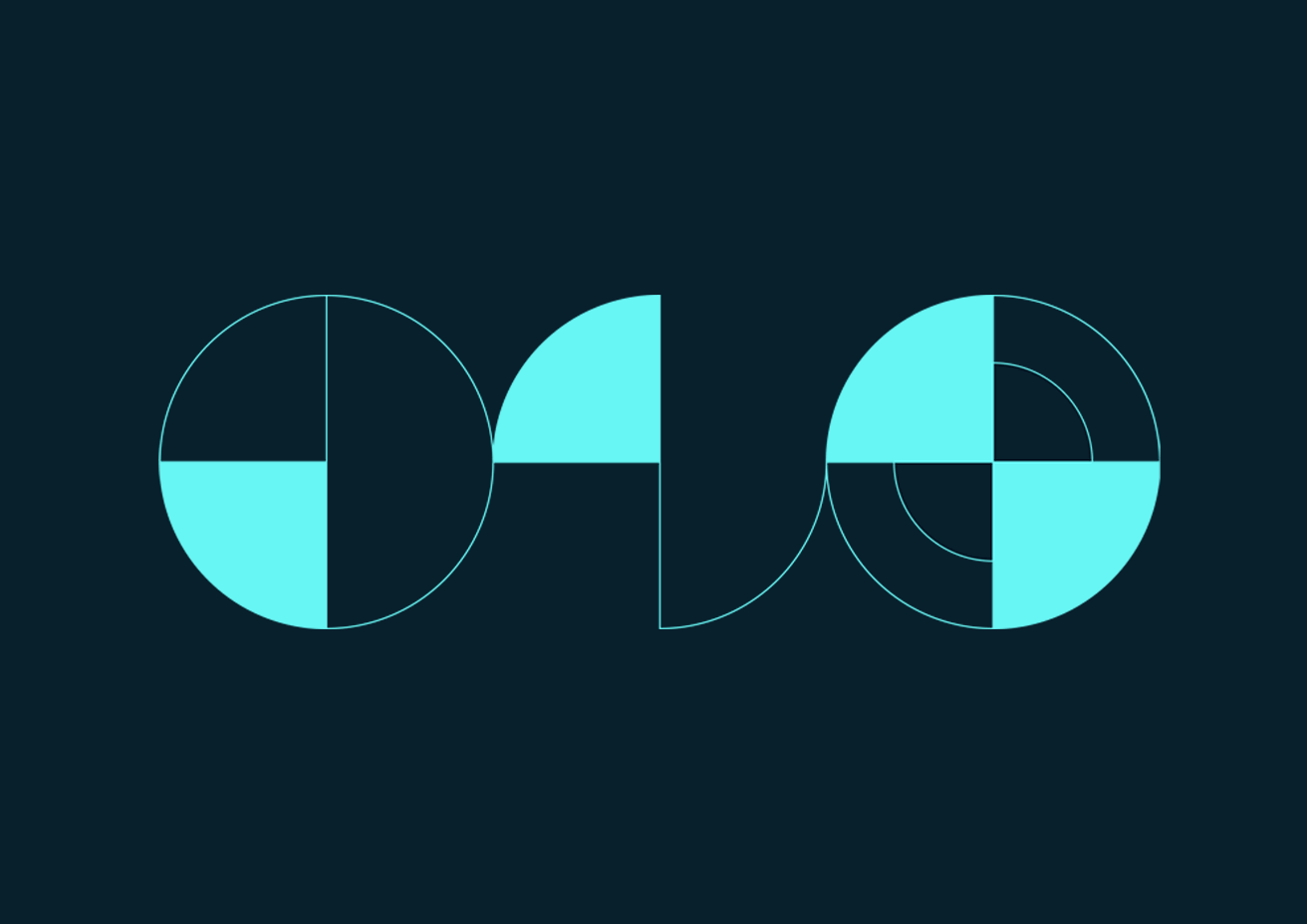
Electric Utility Investment and Maintenance Planning Using Advanced Analytics
Avoid Costly Downtime with Effective Maintenance and Replacement Strategies
For electric utility organizations, prioritizing maintenance and replacement strategies across fleets of complex assets is critical to avoiding costly downtime. But without the right combination of subject matter expertise, data connectivity, and analytics tools, these organizations are left with a number of questions and roadblocks.
Roadblocks to Data-Driven Decisions
Today’s utility organizations collect more data than ever before, but that doesn’t mean it’s accessible. Often spread across thousands of assets, these robust data pools are difficult to harness in one place and even more difficult to analyze due to the number of elements (data sources, calculations, assets, cross-functional teams, etc.) required to achieve valuable insights. These obstacles leave organizations to make important CAPEX and OPEX decisions based only on reactive diagnostics.
With Seeq, these organizations can connect to data from an extensive set of data storage platforms, including historians, SQL databases, and more, to provide a single, accessible location for data manipulation and visibility across assets. This enhanced situational awareness enables more intuitive decision-making around CAPEX, OPEX, and more.
The use cases below illustrate a few examples of how Seeq addresses these roadblocks for electric utility organizations.
Use Case: Solar Panel Tracking/Soiling Losses
In some deployed PV generating plants, solar panels are mounted on motorized single or dual axis tracking structures that adjust panel direction to better absorb solar irradiation and produce more electricity. Over time, environmental conditions like dust, dirt, and rain “soil” the panels, requiring periodic cleaning to avoid degrading the electricity production over time. However, quantifying losses related to axis tracking faults and panel soiling while monitoring a PV generation plant can be difficult to do. Thus, it can be difficult to determine when, from a monetary standpoint, to perform maintenance on the panels.
In this use case, Seeq was used to build a model of solar power generation based on solar irradiance during optimal periods. To create the model, a period during which the panels were clean and trackers were running close to set point was identified. This period was then used to build a linear model of power produced as a function of solar irradiance. Finally, the model of power production was used alongside the tracker angle signal to determine the total power and revenue lost due to tracker angle deviations and panel soiling. This tabular view of daily losses can then be utilized to better estimate when panel cleaning or axis tracking repair should be performed based on the monetary impact to operations rather than a time interval.
Challenge
- Losses due to panel soiling or tracker faults are difficult to quantify
Solution
- Identify periods when each tracker in a block was deviating from set point
- Train a model when panels were clean and trackers were on set point
- Utilize model to determine system losses
Results
- Generated models allow losses related to soiling and axis tracking faults to be calculated and converted to dollar amounts in near real-time
- Action can be taken to clean panels or fix/tune tracking controllers when the cost of loss is greater than the cost of maintenance
Use Case: Weather Based Load Predictions
Monitoring and predicting weather conditions is an essential part of maintaining electrical utility assets, such as rooftop refrigeration units. In order to determine how your system may operate in different weather conditions and, ultimately, avoid downtime from these conditions, organizations must develop electrical load forecasts based on future weather forecasting. This requires integrating on-premise data sources with cloud-based weather forecasts. However, seamlessly moving between historical data and future data is complex, as future data is constantly subject to change.
In this use case, Seeq was used to connect to the NOAA weather forecast to access future data, including wind speed and temperature, and integrate it directly with historical on-premise data to create a predictive model. Knowing that future weather forecasts are often inaccurate, engineers can apply a cone of increasing uncertainty to project the possible range of future values. Running the model allows engineers to view predicted loads for specific assets and gain insights into how they will operate during different weather conditions.
Challenge
- Integrating weather forecasts into asset models to predict future performance
- Integrating disparate data sources to create a unified solution with continuous results
Solution
- Integrate NOAA weather forecasts or OSIsoft Future Data with regression models on historical data to create continuous load predictions
- Model error in forecasts to visualize the range of possible outcomes
Result
- Engineers and operators can quickly spot oncoming problems and implement mitigation strategies or identify opportunities to take advantage
How to Protect Electric Utility Investments
Seeq is the only advanced analytics application specifically designed for process manufacturers and their data. Electric utility organizations can use Seeq to connect disparate data sources and quickly apply industry-standard modeling techniques across assets to identify problem equipment and quantify the value of different replacement or mitigation strategies.
To learn more about Seeq, schedule a live demo today or download this webinar to see these use cases in action.